In today’s data-centric world, “predictive analytics” has become increasingly ubiquitous. But what is predictive analytics, and why is it so crucial across industries? Let’s unravel the power and potential of predictive analytics, exploring its definition, importance, and real-world applications.
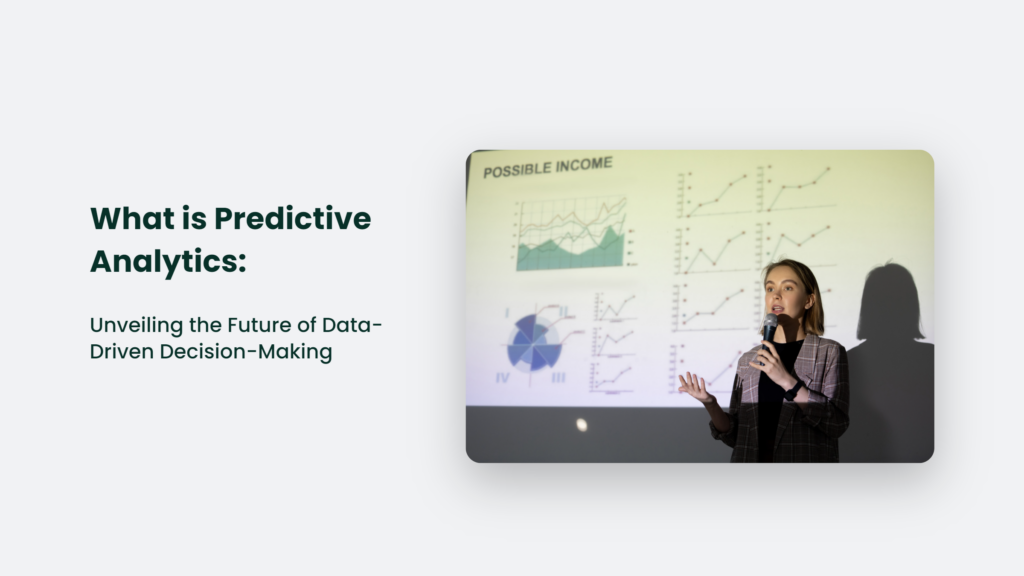
What is Predictive Analytics?
At its core, predictive analytics uses data to forecast potential scenarios and trends, enabling organizations to make informed decisions and develop proactive strategies. It leverages historical data, statistical algorithms, and machine-learning techniques to identify the likelihood of future outcomes based on historical data patterns.
The Essence of Predictive Analytics
The essence of predictive analytics lies in its ability to accurately predict future occurrences, allowing organizations to make critical decisions based on data. By answering the question “What might happen in the future?” predictive analytics empowers businesses to anticipate market trends, optimize processes, and enhance their strategies. This predictive capability enables all business users to make smarter decisions backed by facts, ultimately improving productivity and increasing the team’s confidence.
Predictive analytics involves a systematic flow, starting with identifying the problem to be solved and the project scope. It then progresses to data preparation, model building, and refinement, culminating in applying the predictions to drive positive outcomes. This process is underpinned by using historical data, machine learning, and artificial intelligence to forecast future outcomes, thereby shaping the future of business strategy and decision-making.
The Power of Data-Driven Insights
Data analytics, encompassing descriptive, diagnostic, prescriptive, and predictive analytics, equips organizations with the information necessary to make impactful business decisions. Predictive analytics, in particular, holds the key to unlocking the future, enabling businesses to anticipate and prepare for what lies ahead.
Real-World Applications
From predicting customer churn to forecasting demand, predictive analytics applications are diverse and far-reaching. For instance, in-app marketing and predictive analytics enable marketers to harness the power of AI and machine learning to estimate specific outcomes, thereby enhancing their marketing strategies.
Common Techniques used in Predictive Analytics:
Some common techniques used in predictive analytics include:
- Decision Trees: Decision trees are a popular predictive modelling technique that involves mapping out decisions and their possible consequences. This technique is widely used in various industries, such as finance and healthcare.
- Neural Networks: Neural networks, a subset of machine learning, are used to recognize patterns and interpret data. They are particularly effective in image and speech recognition, as well as in financial forecasting.
- Regression: Regression analysis is a statistical method used to examine the relationship between two or more variables. It is commonly employed in finance, economics, and the natural sciences to make predictions.
- Machine Learning: Predictive analytics is closely tied to machine learning, which involves training algorithms to make predictions based on data. This technique is widely used in fraud detection, marketing optimization, and inventory management.
- Data Mining: Data mining is discovering patterns in large data sets. It is a fundamental technique in predictive analytics, enabling organizations to extract valuable insights and make informed predictions.
Examples of Successful Predictive Analytics Implementations:
Some examples of successful predictive analytics implementations across various industries include:
- Retail: Predictive analytics predicts customer behaviour and buying patterns, enabling retailers to optimize inventory, personalize marketing, and enhance the overall customer experience. For instance, Walmart utilizes predictive analytics to understand buying behaviour and personalize customer interactions.
- Healthcare: In the healthcare industry, predictive analytics detect illnesses, forecast patient outcomes, and improve resource allocation. It helps identify at-risk patients, optimize treatment plans, and enhance healthcare delivery.
- Finance: Predictive analytics is widely used to detect fraudulent financial transactions, predict market trends, and assess credit risk. Financial institutions can make informed decisions and mitigate potential risks by analyzing historical data and patterns.
- Marketing: Predictive analytics is leveraged to forecast customer lifetime value, optimize marketing campaigns, and personalize customer interactions. It enables businesses to target the right audience with the right message at the right time, ultimately driving higher conversion rates and ROI.
- Manufacturing: In the manufacturing sector, predictive analytics is utilized for predictive maintenance, demand forecasting, and quality control. By analyzing historical data and equipment performance, manufacturers can optimize maintenance schedules, reduce downtime, and improve overall operational efficiency.
These examples demonstrate the diverse and impactful applications of predictive analytics across industries, highlighting its role in driving informed decision-making, mitigating risks, and enhancing operational performance.
Embracing Predictive Analytics: A Strategic Imperative
As the global predictive analytics market continues to surge, with an estimated annual revenue of nearly $11 billion, it’s evident that organizations are increasingly recognizing the transformative potential of predictive analytics. By embracing this data-driven approach, businesses can gain a competitive edge and stay ahead of the curve in today’s dynamic and fast-paced landscape.
The Path to Predictive Excellence
To harness the full potential of predictive analytics, organizations must adopt a disciplined data approach, ensuring robustness and accuracy in their predictive models. It involves evaluating and validating predictive models with alternate data sets and creating a data-driven culture to generate the data needed for accurate predictions.
Frequently Asked Questions:
What are the key types of data analytics?
Data analytics comprises descriptive, diagnostic, prescriptive, and predictive analytics. Each type serves a distinct purpose, from summarizing historical data to anticipating future outcomes.
How can predictive analytics benefit businesses?
Predictive analytics can empower businesses to make proactive, data-driven decisions, anticipate market trends, optimize processes, and enhance marketing strategies, ultimately driving growth and innovation.
The Bottom Line:
In conclusion, predictive analytics is not just a buzzword; it’s a strategic imperative for organizations seeking to thrive in the digital age. By leveraging the power of data-driven insights, businesses can unlock the potential of predictive analytics, gaining a competitive edge and charting a course for future success. So, are you ready to embrace the future with predictive analytics?